4 min read
Banks Need Artificial Intelligence – It’s Just a Matter of Picking the Right Technology
By: John Meyer on Jul 13, 2023 10:07:00 PM
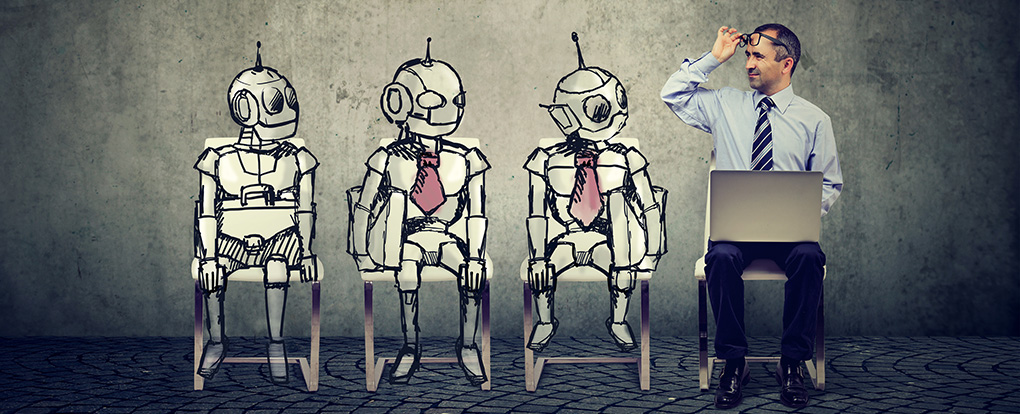
Vendors need to be able to explain their AI technology to bankers in layman’s terms
“An algorithm is what software engineers call something when they don’t want to explain what they did.” —Modern Adage
Every vendor these days is talking about how their software has magical “AI” algorithms in it. How do banks and credit unions choose the applications that are right for their institutions?
There are three generally accepted categories for artificial intelligence today: machine learning, robotic process automation, and natural language processing/generative text. The following definitions and use cases for the application of AI are meant to help bankers push their vendors to explain their technology in layman’s terms.
Machine Learning AI
Machine learning is the type of artificial intelligence that uses a model to predict an outcome. Most data scientists break models into supervised machine learning and unsupervised machine learning.
Supervised machine learning is when a model is trained to predict outcomes from a dataset. Consumer loan decision engines are a classic example of supervised machine learning. These engines look beyond simple credit scores and debt-to-income ratios to determine a new pool of potentially approved loans. Many of these models use utility, telecom, and rent payments along with payroll data to determine a loan applicant’s ability to repay. With their focus on consumer lending, credit unions are especially interested in this technology. Zest.ai and Scienaptic signed more than 80 new credit unions in the past year.
Whether or not banks and credit unions know it, they are using unsupervised machine learning in many of their anti-money laundering and fraud software applications. Verafin, NICE Actimize, and Abrigo all have embraced unsupervised machine learning to detect fraudulent transaction patterns to enhance their behavioral and rules-based detection algorithms.
However, in both supervised and unsupervised machine learning, bankers need to keep in mind that models develop biases that might not comply with things like fair lending and Community Reinvestment Act regulations. As my mother used to ask me, “If all your friends jumped off a bridge, would you?” Machine learning would jump just as quickly as it might determine that race, gender, or ZIP code are important variables to weigh into the decision criteria. Therefore, a vendor that is excited to talk about its machine learning software should be able to explain whether the model is transparent with how it arrived at this conclusion. Can the software “show the math?”
Robotic Process Automation AI
We speak frequently with clients that have already rolled out robotic process automation. RPA is akin to artificial intelligence that “does” rather than “learns” tasks. Banks are embracing tools like Pega, UI Path, Microsoft’s Power Automate, and Nintex to create automated workflows for loan processing and other tasks that a human would manually do. One bank I spoke with has automated the fraud case investigation process to create a dossier on a subject by automatically searching negative media, Google, LinkedIn, and other social media outlets so that fraud investigators can cut their research time down to just minutes per alert.
Natural Language Processing/Generative AI
While bankers we talk with are excited about how natural language processing/generative AI can be used to enhance their service offerings, some are wary of introducing engines like ChatGPT without further guidance from the regulators. However, we are hearing that more and more institutions are using these engines to create internal knowledge centers where their teams can get policy, procedure, or basic referral information. There is even a tutorial online that shows how someone can use ChatGPT to create tailored LinkedIn messages and “coffee chat” questions. Hmm, we wonder if some of the commercial loan officers who are looking for new opportunities have seen that video.
AI is already here in the banking sector. Bankers just need to determine which AI applications will help their institutions continue to grow while managing risk.
Author’s note: We would love to hear how financial institutions are executing AI initiatives at their institutions. Please share a brief example in the comment box below.
5 min read
The Holy Quest for CRM in Anytown, USA
Apr 25, 2024by Elizabeth Gujral
7 min read
Where Does Chime Go From Here?
Apr 4, 2024by Ron Shevlin